From the lab to the patient
The number of possible drug-like molecules can baffle the imagination. There are more than 10⁶⁰ compounds in this molecular haystack, though only a tiny proportion of these compounds will meet all the requirements to become a safe and effective medicine. Over the past millennia, we found a handful of needles (or cures) in this molecular haystack. More often than not, we have relied on serendipity, such as Fleming’s discovery of penicillin.
There are more than 10⁶⁰ compounds in the molecular haystack, though only a tiny proportion of these compounds will meet all the requirements to become a safe and effective medicine.
As we venture into fighting more complex diseases, we cannot rely on chance alone. Building on enormous advances in chemistry and biology over the past 100 years, we have developed systematic approaches towards discovering molecules with therapeutic properties. This way, a standard model of drug discovery has emerged over the past decades. First, we identify a target, i.e. a piece in the biological machinery — usually a gene or a protein as a gene-product — which is hypothesised to play an essential role in a specific disease. Then, researchers explore vast chemical libraries in a process called high-throughput screening to find hits, chemicals that show an effect on the target of interest. These hits are then shortlisted and form the starting point to develop a lead molecule (which over time can become a new drug). A lead compound will have been optimised for months or years, to find the best possible therapeutic effect and the lowest possible risk.
Still, a long path lies ahead for any given lead candidate. First of all, a compound with promising results in cells or even mice might not have the desired effect in humans. Even worse, compounds can have damaging, if not lethal, consequences. For example, in 1961, the newly developed sedative thalidomide was found to cause severe congenital disabilities and even death in babies when their mothers took the drug early in their pregnancies. This disaster led countries around the world to update their regulatory policies concerning drug development. Now, every drug must follow an established regulatory drug development pathway, including extensive testing in both preclinical and clinical settings, to ensure that only safe and effective drugs finally reach patients (Fig. 1).]
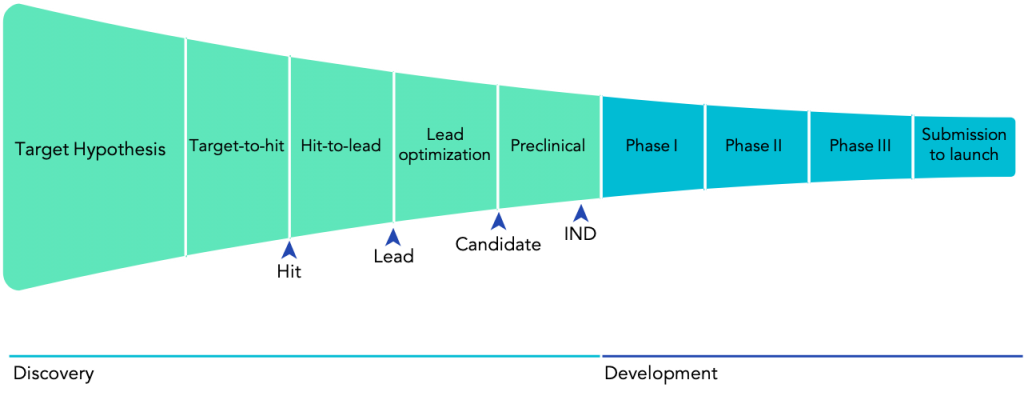
Turning the tables on Eroom’s law
Since the 1950s, most industries and parts of human life have experienced a significant boost in productivity thanks to advances in computing and automation. Underpinning most of this progress is Moore’s law which describes how transistor density on microchips roughly doubles every 18 to 24 months. So far, this prediction has held for over six decades, driving productivity to unprecedented levels. In stark contrast, the productivity of the drug development industry has followed the opposite trend. This has led to the emergence of the term ‘Eroom’s Law’ — Moore’s Law in reverse — that states that the cost of R&D to bring a new drug to FDA approval is doubling roughly every nine years. Now, it takes a staggering $2.6bn dollars and around 15 years to bring a single new drug to the market.
Eroom’s law results from the interplay of diverse factors. It is rooted as much in scientific challenges as economic incentives. Nonetheless, it is clear that the drug discovery and development processes have not seen the same transformation that other industries have experienced.
The cost of R&D to bring a new drug to FDA approval is doubling roughly every 9 years. It takes a staggering $2.6bn dollars and around 15 years to bring a single new drug to the market.
For example, the adoption of automated workflows has been relatively slow in drug discovery. Still today, the overwhelming majority of the critical experimental work in drug discovery relies on error-prone manual procedures. However, over the past years, we have witnessed huge leaps in robotic technology for biopharmaceutical applications, and robotics are almost within life scientists’ reach. Access to remote automated laboratories such as Arctoris’, and user-friendly human-machine interfaces like ChemOS and LabView, are offering to scientists the kind of tools that have been accelerating the work of engineers for decades. Automation opens the opportunity to reduce human error, create rich, machine-readable datasets, and free scientists’ time to be spent on the tasks that truly require human creativity and ingenuity.
At the same time, during the last decade, computational advances reached the threshold where they can have a transformative impact on drug discovery and development. Consider the fundamental question of how a protein folds into the three-dimensional shape that determines its function. Breakthroughs in artificial intelligence, specifically new advances in deep learning, culminated in the groundbreaking results achieved by DeepMind’s AlphaFold 2 engine last month (Fig. 2). With these types of tools, it is imaginable that it will soon be possible to predict the full structure of a protein with the click of a mouse.
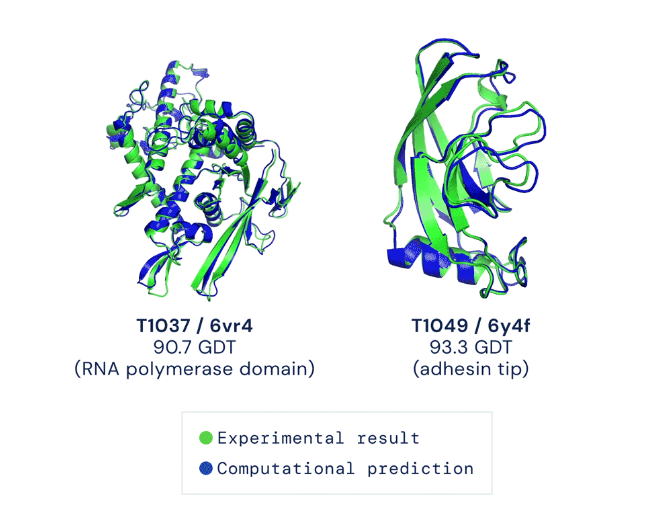
Source: The AlphaFold Team. “AlphaFold: a solution to a 50-year-old grand challenge in biology.” Deepmind.
Upgrading the drug discovery process with AI techniques and robotics will have an enormous impact on the whole ecosystem. The discovery phase of a new drug takes between 4 and 5 years on average and amounts for almost one-third of the cost of bringing a drug to the market. Further, drug discovery is confronted with both the problem of false positives and false negatives, both of which can become costly mistakes. In the end, candidates often only fail in Phase II trials (Fig. 1), in 2 out of 3 cases due to lack of efficacy or unexpected toxicity concerns. Failures at such an advanced stage are extremely costly both in terms of money and time.
The discovery phase of a new drug takes between 4 and 5 years on average and amounts for almost one-third of the cost of bringing a drug to the market.
Improvements in the discovery stage will directly lead to improvements downstream. First of all, robotics and AI can drastically shorten cycle times. This was impressively demonstrated last year by Hong Kong-based Insilico Medicine. Relying on novel machine learning techniques, they sped up the generation of lead candidates from the pharma average of 1.8 years to just 45 days. This type of approach, coupled with 24/7 automated experimental execution, could reduce cycle times across the entire drug discovery process. Further, automation fosters the capture of rich and reliable data and metadata, which feeds high-quality input data to AI algorithms and improves their performance.
Bringing the power of AI and automation to bear on the early parts of the drug discovery funnel will radically change its shape by expediting the early phases and filtering out potential failures promptly. With these tools, we will get a chance to move from Eroom’s to Moore’s law in drug discovery.
To learn more about how drug discovery can be transformed by AI and automation, watch the Arctoris-Goodwin Drug Discovery Masterclass on-demand here:
- ‘From bench to bedside’ by Martin-Immanuel Bittner MD, CEO and Co-founder, Arctoris
- ‘An introduction to early-stage drug discovery and the importance of high-quality, reproducible data’ by Daniel Thomas PhD, Head of Discovery Biology, Arctoris