How automation can help researchers document experiments
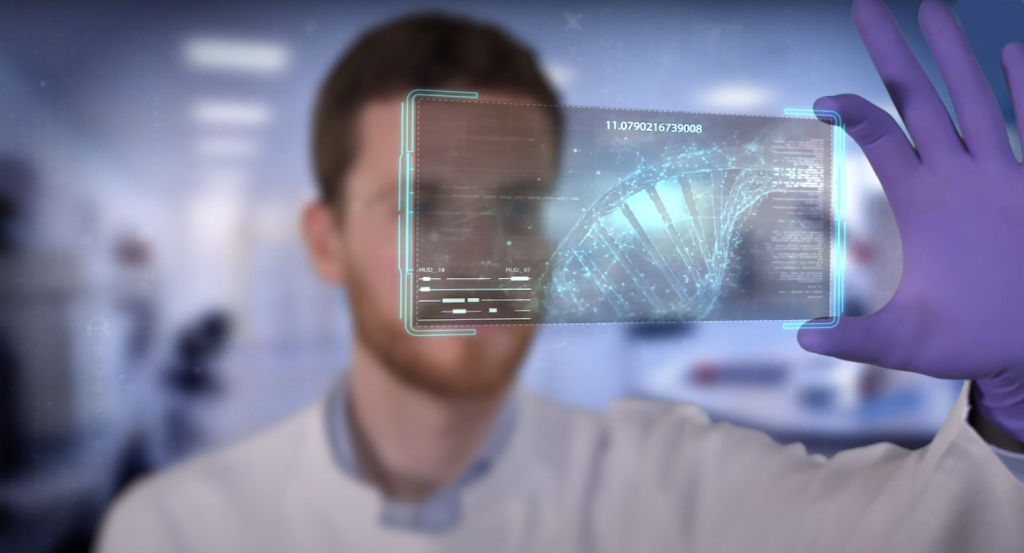
Scientists and lab technicians have to meticulously follow scientific protocols to ensure the validity of their findings. Certain parts of lab work lend themselves to effortless measurement, making them easy to describe in the methods section of protocols and research papers (e.g. volumes and concentrations). Then, there are those details of the workflow that are too tedious to record, such as temperature fluctuations or the exact timings of every single experimental step. Finally, there are descriptions written using vague terminology. Ambiguous descriptions are surprisingly common in the scientific literature; for example, during the first half of 2020 alone, the phrase “shake gently” was used over 3000 times in peer-reviewed research articles.
A “gentle shake” might be interpreted in a variety of ways, and each will lead to slightly different experimental results. A richer description of the same step would include information about the type of motion, intensity, timing, temperature, frequency etc [1]. This is just one of many instances in which the lack of metadata — information about the creation, context, and content of data used in research [2] — impacts research studies and hinders its reproducibility.
During the first half of 2020 alone, the phrase “shake gently” was used over 3000 times in peer-reviewed research articles.
Without reliable metadata, researchers that want to replicate research studies cannot do so. This challenge is a key driver of the reproducibility crisis, casting doubt on large parts of published literature. This concern is particularly troubling in basic biomedical research, which forms the foundation of drug discovery efforts. Current estimates for the percentage of irreproducible preclinical research stand at 80 to 90%, costing as much as $28.2 billion per year in the US alone [3]. Among the key reasons contributing to this problem are well-known issues concerning the validity of biological reagents, protocol adherence, rigour and impartiality of data analysis, and reliability of data storage and retrieval. All of these can be improved by employing standardised scientific processes and complete data capture including all metadata.
Current estimates for the percentage of irreproducible preclinical research stand at 80 to 90%, costing as much as $28.2 billion per year in the US alone.
Recently, a study evaluated the quality of metadata for a collection of more than 11m biological samples and revealed serious anomalies [4]. The majority of metadata fields were neither standardised nor controlled. Non-compliant values were found even in simple binary fields. This issue has become even more concerning during the ongoing SARS-CoV-2 pandemic, in which the haste to publish new findings has led to metadata not being reported in sufficient depth and detail at an even larger scale [5]. These omissions are a severe obstacle when trying to reproduce and thus validate new hypotheses; in addition, they make it impossible for datasets to be successfully analysed using computational tools or machine learning techniques. These techniques could be used to discover patterns that evade scientists, but they are heavily reliant on the quality and annotation of the input data.
The scientific community, including top scientific journals and research agencies, have launched several initiatives to improve reporting on data collection and handling to boost reproducibility. The leading academic journal Nature published an 18-point checklist [6] which guides researchers in paying greater attention to metadata and statistical information. Another commendable effort is the introduction of FAIR Guiding Principles for scientific data management and stewardship. FAIR stands for Findability, Accessibility, Interoperability, and Reusability each being a key concept in data collection and storage ensuring that data can be re-used in future research and also analysed computationally [7]. Without such efforts, failure rates in drug discovery will remain unacceptably and unsustainably high.
Alas, human reporting alone will never be encompassing enough to meet these requirements. However, automation can change this. With the aid of automation, conducting experiments in a precise way while capturing rich and robust metadata suddenly becomes possible, simultaneously releasing precious time in researchers’ busy workdays. Automated platforms can easily capture in-depth metadata for a multitude of experimental conditions such as reaction times, temperature and humidity etc. Furthermore, robotic experiment execution requires clear, unambiguous instructions, encouraging the creation of precise and detailed protocols. This precision pays off, as full audit trails can automatically be generated, allowing for the complete replication of experimental conditions at the click of a button.
To overcome the limitations of metadata capture and documentation performed by researchers and lab assistants, Arctoris offers storage of project data and experiment metadata in a standardised, FAIR-compliant format. The automated metadata collection is illustrated in Figure 1, where a conventional biochemical IC50 assay is juxtaposed with the same assay generated in a fully automated setting. Not only is the data density more than two orders of magnitude higher, but the robotic platform also provides the complete, detailed, time-stamped context of the experiment with continuous monitoring of temperature and humidity levels.
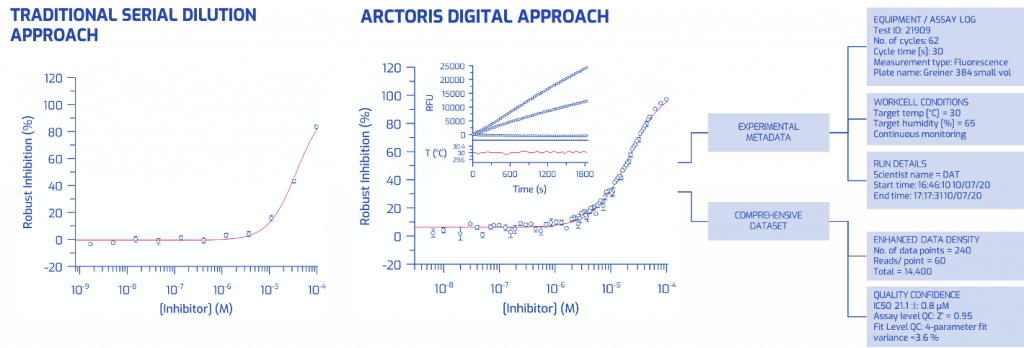
Now that scientists can pass on the grunt of experimental execution to robots, they can finally do what they are best at: project oversight, experiment planning, data interpretation, communication and discussion of findings; in other words, genuine scientific work.
References
- May, M. The Many Ways to Shake Samples. Lab Manager https://www.labmanager.com/product-focus/the-many-ways-to-shake-samples-2880 (2020).
- Best practice data life cycle approaches for the life sciences. https://www.ncbi.nlm.nih.gov/pmc/articles/PMC6069748/.
- Freedman, L. P., Cockburn, I. M. & Simcoe, T. S. The Economics of Reproducibility in Preclinical Research. PLOS Biol. 13, e1002165 (2015).
- Raphael, M. P., Sheehan, P. E. & Vora, G. J. A controlled trial for reproducibility. Nature 579, 190–192 (2020).
- Gonçalves, R. S. & Musen, M. A. The variable quality of metadata about biological samples used in biomedical experiments. Sci. Data 6, 190021 (2019).
- Kiermer, V. Reporting Checklist. (2015).
- Wilkinson, M. D. et al. The FAIR Guiding Principles for scientific data management and stewardship. Sci. Data 3, 160018 (2016).